Generative Zoo
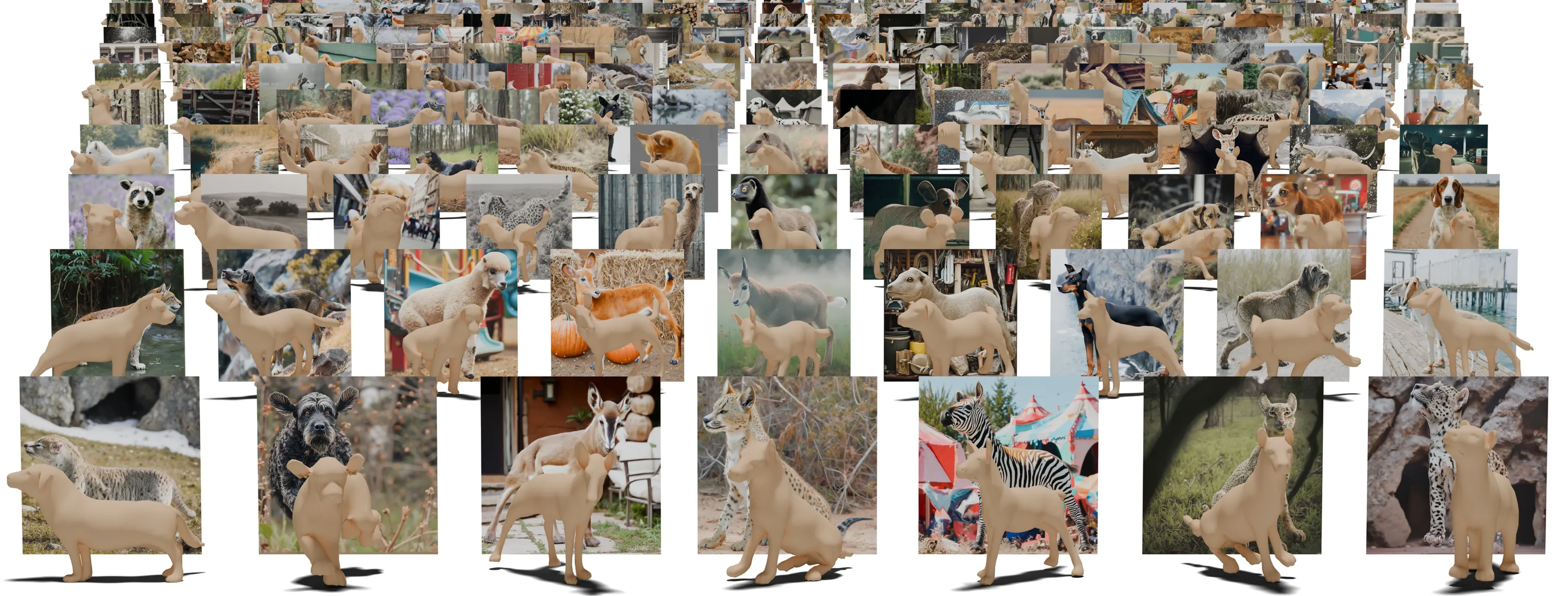
Summary
We propose a pipeline for the scalable generation of realistic 3D animal pose and shape estimation training data. Training solely on data produced using our pipeline, we achieve state-of-the-art performance on a real-world 3D pose and shape estimation benchmark.
Goal
To reconstruct animals in the wild in 3D from monocular images.
Problem
It is difficult to obtain training data that is both accurately annotated and realistic.
Some have attempted to address the challenge of procuring training data by pseudo-labeling individual real-world images through manual 2D annotation, followed by 3D-parameter optimization to those labels. While this approach may produce silhouette-aligned samples, the obtained pose and shape parameters are often implausible due to the ill-posed nature of the monocular fitting problem.
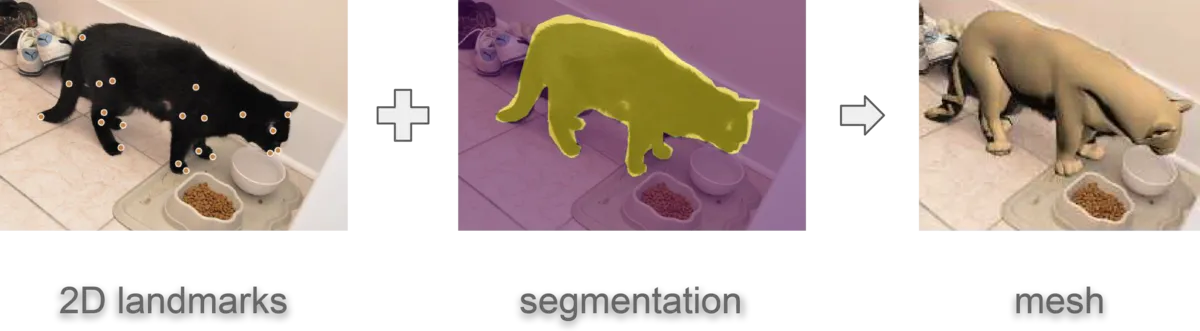
Sidestepping real-world ambiguity, others have designed complex synthetic-data-generation pipelines leveraging video-game engines and collections of artistdesigned 3D assets. Such engines yield perfect ground-truth annotations but are often lacking in visual realism and require considerable manual effort to adapt to new species or environments.
Solution
We investigate a potentially simpler alternative to the production of synthetic data: rendering with a conditional image-generation model. We propose a pipeline that, given the name of a species, produces paired images and groundtruth pose-and-shape parameters. Our pipeline facilitates the generation of realistic images with a degree of control comparable to traditional synthetic-data generators, thus combining the advantages of visual realism, scalability, and controllable data production.
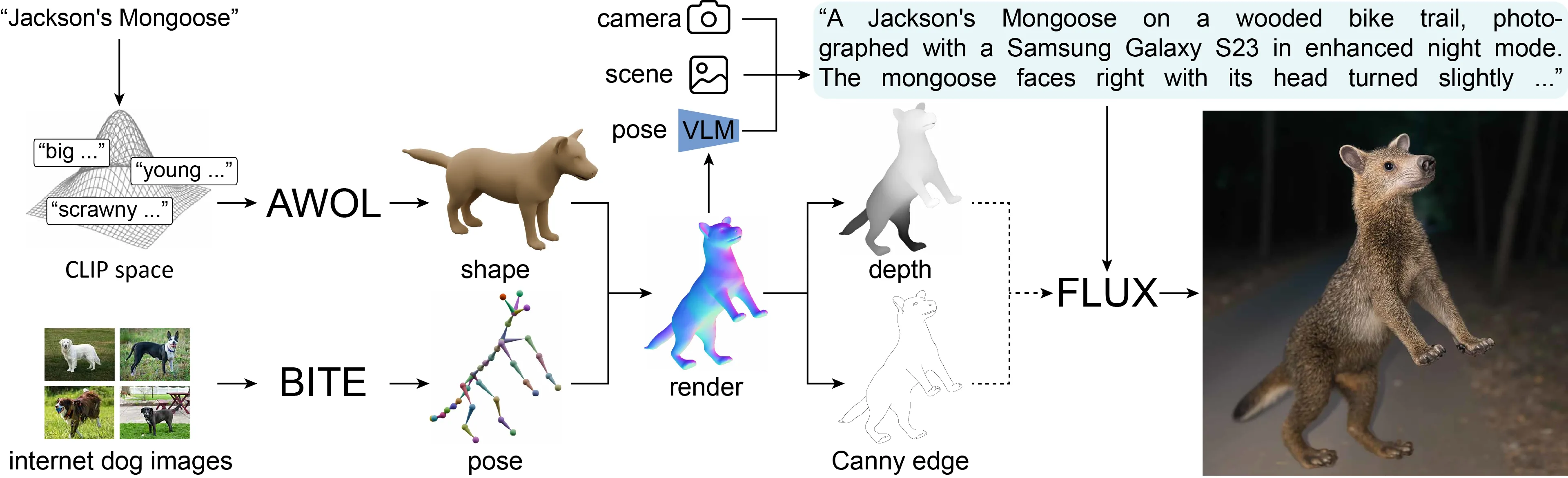
Dataset
To demonstrate the scalability of our approach, we introduce GenZoo, a million-image dataset comprised of unique poses and shapes across diverse mammalian quadrupeds.
Model
Training a regression model solely on our synthetic dataset, without the use of ground-truth real-world images, we achieve state-of-the-art performance on Animal3D, a real-world animal pose and shape estimation benchmark, validating the quality of our dataset.
Paper
The model-based estimation of 3D animal pose and shape from images enables computational modeling of animal behavior. Training models for this purpose requires large amounts of labeled image data with precise pose and shape annotations. However, capturing such data requires the use of multi-view or marker-based motion-capture systems, which are impractical to adapt to wild animals in situ and impossible to scale across a comprehensive set of animal species. Some have attempted to address the challenge of procuring training data by pseudo-labeling individual real-world images through manual 2D annotation, followed by 3D-parameter optimization to those labels. While this approach may produce silhouette-aligned samples, the obtained pose and shape parameters are often implausible due to the ill-posed nature of the monocular fitting problem. Sidestepping real-world ambiguity, others have designed complex synthetic-data-generation pipelines leveraging video-game engines and collections of artist-designed 3D assets. Such engines yield perfect ground-truth annotations but are often lacking in visual realism and require considerable manual effort to adapt to new species or environments. Motivated by these shortcomings, we propose an alternative approach to synthetic-data generation: rendering with a conditional image-generation model. We introduce a pipeline that samples a diverse set of poses and shapes for a variety of mammalian quadrupeds and generates realistic images with corresponding ground-truth pose and shape parameters. To demonstrate the scalability of our approach, we introduce GenZoo, a synthetic dataset containing one million images of distinct subjects. We train a 3D pose and shape regressor on GenZoo, which achieves state-of-the-art performance on a real-world 3D animal pose and shape estimation benchmark, despite being trained solely on synthetic data. We will make public our dataset and data-generation pipeline to support future research.
Extended Visuals
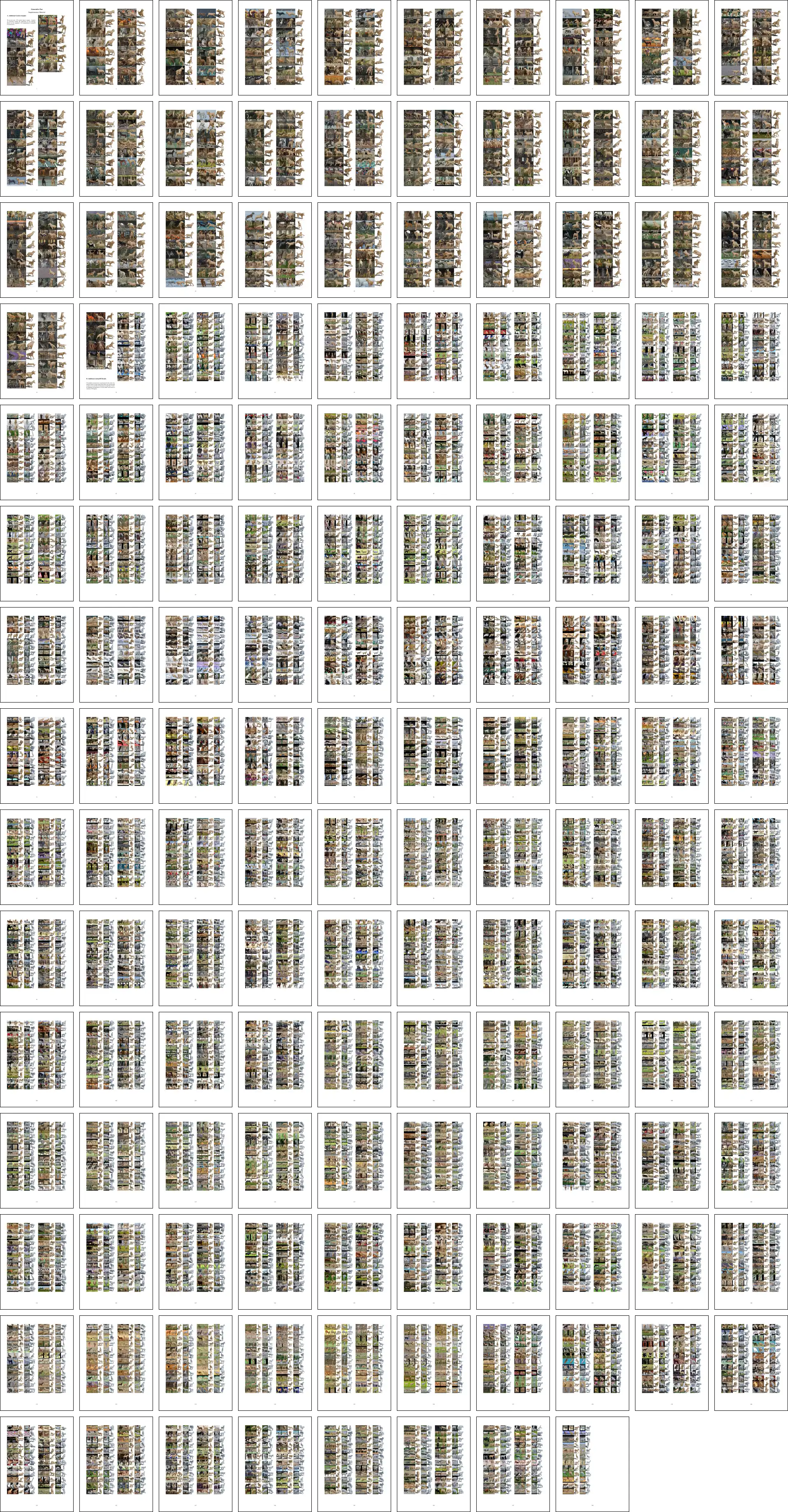